With Gary Collier, CTO of Man Group Alpha Technology, and Hinesh Kalian, Director of Data Science, Man Group
What are the main data challenges / pain points for the buy side?
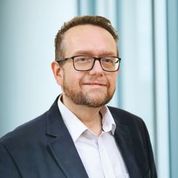
A big challenge is obtaining and retaining data science talent. It is apparent that there is a growing demand, and therefore competition, for data science talent across all industries, not just in financial services. Another challenge relates to the ability to ingest and curate structured and unstructured data rapidly and in a variety of raw formats. The growth in new data providers has led to a wide variance in the quality of data offered by data providers; some providers are well-established and have appropriate data science and technology teams, whereas others can be as limited as two employees in a start-up.
For data to be useful it needs to be clean, consistent and sourced and processed appropriately. Often data is provided after some processing steps are done, which limits awareness of the raw data and can lead to the risk of false representation and predictability.
How does Man Group leverage data as a competitive advantage?
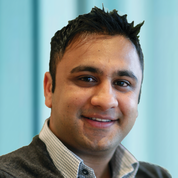
Data is the raw ingredient that fuels alpha models and investment decisions. In order to avoid the garbage-in/garbage-out conundrum, you need to improve your data collection and organization and create consistent methods to cleanse, process and find insights from data.
Man Group’s data science specialized function utilizes advanced technology for rapidly sourcing and on-boarding new data sources and combines quantitative skill sets to filter out the noise, creating a potential competitive advantage in a fast and evolving landscape. These capabilities lead to the democratization of data, breakdown of silos and access to data at scale. As firms continue to add to this scale, you end up with a data “ecosystem” which creates the ability to combine multiple data sources, in multi-dimensional ways, to build alphas and risk models.
To be competitive, we need to look at the broader landscape to efficiently discover new data sources to test investment hypotheses and invest in building leading edge technology that unveils the true potential of the data. Our continued investment in building our data infrastructure and the usage of advanced technologies has provided us with the scale to source, process, store and evaluate vast amounts of big data.
Another dimension to gaining a competitive advantage relates to talent and close ties to academia. At Man Group, our continued focus on academia, technology and open source communities provides our talent pool with diverse ways to further develop their expertise. For example, the Oxford-Man Institute has focused on machine learning and data science over the last decade, connecting our research teams with renowned academics from around the world. Additionally, our data teams are exposed to a mix of quantitative and discretionary investment management styles.
Gary, you have been with Man Group for almost 20 years, how has data science evolved in asset management over that time?
It’s been a very interesting evolution. For the first 17 years of my time at Man, I worked for Man AHL, our quantitative systematic investment Engine. Man AHL, now with a track record of over 30 years, was one of the original practitioners of computer-driven systematic trading. Throughout this history, the key theme has been the analysis of large data sets in order to scientifically test hypotheses of financial market behaviour, and ultimately build automated models to extract these signals and trade based on them. So in a very real sense, Man Group was “doing data science” in asset management long before the term was in common usage.
But what has certainly changed has been the volume and type of data, and the techniques and technologies needed to analyse them and extract value. The world of 20 years ago typically involved application of statistical techniques using custom technology environments (built in-house using low-level languages such as C (a structured oriented programming language) to fundamental data, or simple time-series data such as asset price or traded volume. Nowadays, elements that contribute to the “objective truth” about the value of an asset exist in a multitude of different data sources, many of which are not necessarily numerical, are large in size and contain high levels of noise. Technology has had to advance on all fronts, from low-level compute, storage and network infrastructure to deal with the increased scale of the problem, to harnessing the huge data science power inherent now in the ecosystem which surrounds the Python programming language.
Analysis techniques have also evolved. Statistical and fundamental analysis are, of course, still key. But these traditional techniques have been augmented with the likes of machine learning, natural language processing and even image analysis.
Organisationally, we also see data science emerging as a first-class concern and department in its own right at many asset managers, and Man Group is no exception. Building a specialised function which draws together a range of quantitative and operational skills, including the ability to scout new sources of data, use advanced technology for rapid on-boarding, perform initial value-add analysis and deal with what is often a “dirty/noisy” space. A combination of all of these skills is necessary if you want to stay at the forefront of a field which is both fast-evolving and constantly producing new data sets.
What kinds of backgrounds do you look for in new hires? Gary has a degree in Theoretical Physics, a STEM background that I imagine was unusual in the industry in the 1990s, but perhaps that’s more the norm today?
There seems to be no specific golden pool, however, recruiting talent that have strong academic backgrounds, Masters or PhDs in various fields like physics, computer science, statistics, finance and machine learning has worked well. Candidates who are likely to have a passion for data, curiosity, enjoy scrutinizing data and have strong academic foundations will likely be in a stronger position. An ideal candidate may wear many hats and must have the ability to communicate insights in the data to the wider audience. We have seen success in employees that are a hybrid of data manipulator, data scientist, engineer and communicator.
Does Man buy and build its data infrastructure? Or just build? What is the rationale for the firm’s approach?
Man Group uses a combination of purchased vendor products, open-source software and in-house software in an attempt to create an overall best-of-breed data platform. For physical infrastructure, whilst we do make use of public cloud, in many ways we favour a contrarian approach, leveraging the performance and end-user experience made possible by our in-house private cloud running on servers, flash storage and networking. We use a very small number of vendor software products, instead leveraging large amounts of open-source software, and combining this with in-house code and “secret sauce” to build high-performance data streaming pipelines, storage and distributed compute and analysis frameworks. Unlike many asset managers, we also contribute extensively to the open-source community in the data space, and have also open-sourced some of our proprietary code including Arctic, a high performance tick and time-series store, and D-Tale, an exploratory data visualisation tool.
What is the future of data science in the buy-side front office? How do businesses stay ahead of the curve?
It is clear that buy-side firms have increased their data spend considerably over the last five years; the number of alternative data full-time employees has grown fourfold over this time period. This directly impacts the demand for superior data acquisition and data science capabilities. The role of data science will become an increasingly prominent function in buy-side firms. Funds will need to build and enhance their infrastructure and data science capabilities to deal efficiently with the vast amounts of data available. Not every new data source will provide value to your portfolio. There is a cost associated with sourcing, testing and evaluating new and alternative data. To stay ahead of the curve, firms need to continue evolving and researching new ideas, invest in data science talent and introduce advanced technologies and solutions to process unstructured data.